In today’s data-driven world, the importance of harnessing the potential of data cannot be overstated. Todd Schneider, a data scientist and engineer, has made significant contributions to this field through his work on what he calls “Medium Data.” In this article, we will delve into the concept of Medium Data, explore Todd Schneider’s contributions, and understand how it can benefit various industries.
Todd Schneider’s Medium Data: Insights and Innovations
Todd Schneider’s recent Meetup event unveiled a fascinating journey through the world of Medium Data, leaving attendees inspired by his remarkable insights and data innovations. During the event, Todd shared a treasure trove of code on GitHub, shedding light on his meticulous data analysis processes. Notably, he tackled the challenge of massive raw data, creatively using an external hard drive to store and efficiently convert it into a SQL database with PostgreSQL. His pragmatic approach demonstrated resourcefulness in managing large datasets.
One intriguing facet of Todd’s presentation was his preference for using R over Python in data analysis. However, it was clear that his expertise transcended programming languages, evident in his clever strategy to reduce the granularity of geocoded events. By working with them as weighted square blocks within New York City, typically measuring around 10 meters by 10 meters, Todd significantly downsized the dataset without compromising analytical depth. For instance, when visualizing “taxicab pickups,” he subdivided the geographic area into smaller boxes, counted pickups within each box, and graphed the results, reducing the dataset size by over a factor of 10.
Todd’s coining of the term “medium data” to describe datasets that can be processed on a personal computer resonated with the audience, reflecting his practical and innovative approach to data analysis. He also shared valuable advice for data enthusiasts, emphasizing the importance of genuine interest in the subject matter to create compelling analyses that resonate with others.
Among the fascinating insights Todd presented was a revealing graph illustrating overall rides in taxis and Ubers. This visual suggested a noteworthy trend—Uber’s growing market share at the expense of traditional taxis. More intriguingly, it indicated that the total number of rides had remained relatively stable, hinting at a zero-sum game. This finding challenged the common perception that Uber contributes to increased traffic, sparking discussions about the broader impact of ride-sharing on urban transportation. Todd Schneider’s Medium Data journey left attendees enlightened and eager to explore the possibilities within this dynamic field.
The Significance of Medium Data
Medium Data stands as a pivotal force in the contemporary data landscape, and its significance transcends conventional boundaries for several compelling reasons:
- Accessibility: One of the most compelling aspects of Medium Data is its accessibility. Unlike the behemoth that is Big Data, Medium Data is well-suited for businesses and organizations that might lack the colossal resources required to navigate the intricacies of massive datasets. It serves as a democratizing force, allowing a broader spectrum of entities, regardless of their scale or financial prowess, to harness the power of data-driven insights;
- Actionable Insights: At the heart of Medium Data’s significance lies its ability to yield actionable insights. These insights are the lifeblood of informed decision-making, guiding strategies and shaping the direction of organizations. Medium Data’s sweet spot between Big and Small Data means that it is expansive enough to provide rich insights, yet not so overwhelming that it hinders the extraction of meaningful information. This makes it a valuable asset for businesses seeking to enhance their decision-making processes;
- Real-Time Analysis: In an era defined by the rapid pace of change, the real-time capabilities of Medium Data stand as a crucial advantage. It facilitates the swift analysis of data streams, enabling organizations to respond promptly to evolving trends and unforeseen events. This real-time aspect can be a game-changer in industries where timeliness is of the essence, such as finance, logistics, and healthcare;
- Cost-Effective: Another notable feature is the cost-effectiveness of Medium Data. Managing the colossal infrastructure and computational resources needed for Big Data can be financially daunting for many organizations. Medium Data, with its manageable scale and computational requirements, offers a more economical alternative without compromising the depth and breadth of data analysis.
Real-World Applications
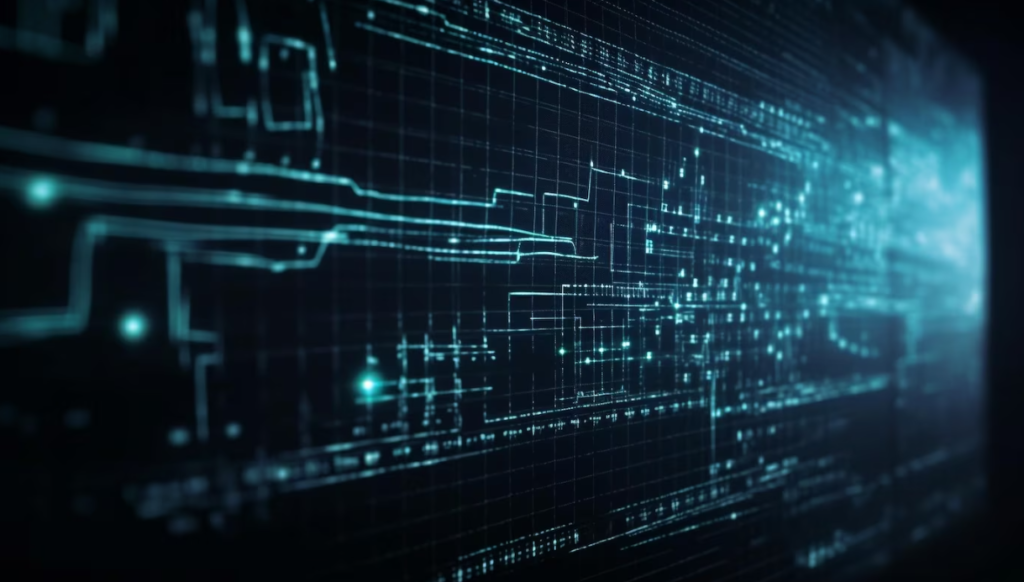
The versatility of Medium Data is evident in its wide array of real-world applications, where its impact extends far beyond the realm of theoretical data science. Across various industries, Medium Data is proving to be a transformative force, driving innovation and reshaping the way businesses and organizations operate:
- E-commerce: Within the sprawling e-commerce landscape, Medium Data takes center stage. By meticulously analyzing user behavior, purchase patterns, and product reviews, businesses can fine-tune their strategies. It allows for the creation of personalized product recommendations, enhancing the overall customer experience. Medium Data’s insights serve as the compass guiding e-commerce platforms toward greater customer satisfaction and improved sales figures;
- Healthcare: In the critical domain of healthcare, Medium Data plays a pivotal role. Patient data, when harnessed and analyzed effectively, can facilitate early disease detection and intervention. The wealth of data available can also be instrumental in optimizing the allocation of hospital resources, ensuring that healthcare facilities are better equipped to meet the needs of their patients. Medium Data’s contributions to healthcare promise to enhance patient outcomes and streamline healthcare delivery;
- Finance: Within the intricate world of finance, Medium Data acts as a discerning eye, capable of identifying nuanced market trends and patterns. This ability is invaluable for investors and financial institutions seeking to make informed decisions. Moreover, Medium Data’s analytical prowess extends to the realm of fraud detection, where it can swiftly identify irregularities and protect against financial threats. It also enables the delivery of personalized financial advice, empowering individuals to make sound financial choices tailored to their unique circumstances;
- Transportation: In the realm of transportation, Medium Data optimizes efficiency and convenience. By analyzing data on routes, traffic patterns, and user preferences, it offers the potential to revolutionize how we move from place to place. Predictive maintenance, a hallmark of Medium Data analytics, ensures that vehicles and infrastructure are maintained proactively, reducing downtime and enhancing safety. User experiences in ridesharing services are also elevated, with data-driven optimizations making journeys smoother, more reliable, and cost-effective.
These are just a few examples of the real-world applications of Medium Data, illustrating its remarkable versatility and potential for transformation. As industries continue to embrace data-driven approaches, Medium Data remains at the forefront, driving innovation and reshaping the way businesses and organizations interact with data to achieve their goals.
Challenges and Considerations
While Medium Data brings a wealth of opportunities, it also presents a set of intricate challenges and considerations that data professionals must navigate with finesse:
- Data Quality: The integrity of data is paramount in the world of Medium Data. Given its diverse origins, ensuring data accuracy and reliability can be a formidable challenge. Data may stream in from various sources, each with its own quirks and idiosyncrasies. Cleaning and harmonizing this data to ensure its quality and consistency become an essential but complex task. Errors or inaccuracies can have cascading effects, potentially leading to misguided decisions based on flawed insights;
- Privacy Concerns: In an age where data privacy is a paramount concern, Medium Data is not exempt from stringent regulations and ethical dilemmas. Handling sensitive data, especially when it pertains to individuals, necessitates strict adherence to privacy regulations and ethical considerations. Organizations must establish robust data governance frameworks to protect individuals’ rights and confidential information. Failing to do so can result in legal repercussions and reputational damage;
- Scalability: As datasets within the Medium Data category continue to accumulate, a challenge emerges—the demarcation between Medium Data and Big Data becomes increasingly blurred. What was once manageable may evolve into a data infrastructure demanding the capabilities of larger-scale data handling. This evolution can strain existing systems and workflows, requiring organizations to adapt with scalable solutions. Preparing for this scalability and the potential shift from Medium to Big Data is a strategic consideration that requires careful planning.
These challenges underscore the need for a holistic approach to Medium Data management. Addressing data quality issues involves meticulous data cleaning and validation processes, while privacy concerns mandate the implementation of robust security measures and privacy compliance. Scalability considerations require organizations to be agile, ready to evolve their data infrastructure and analysis methodologies as data volumes grow.
The Future of Medium Data
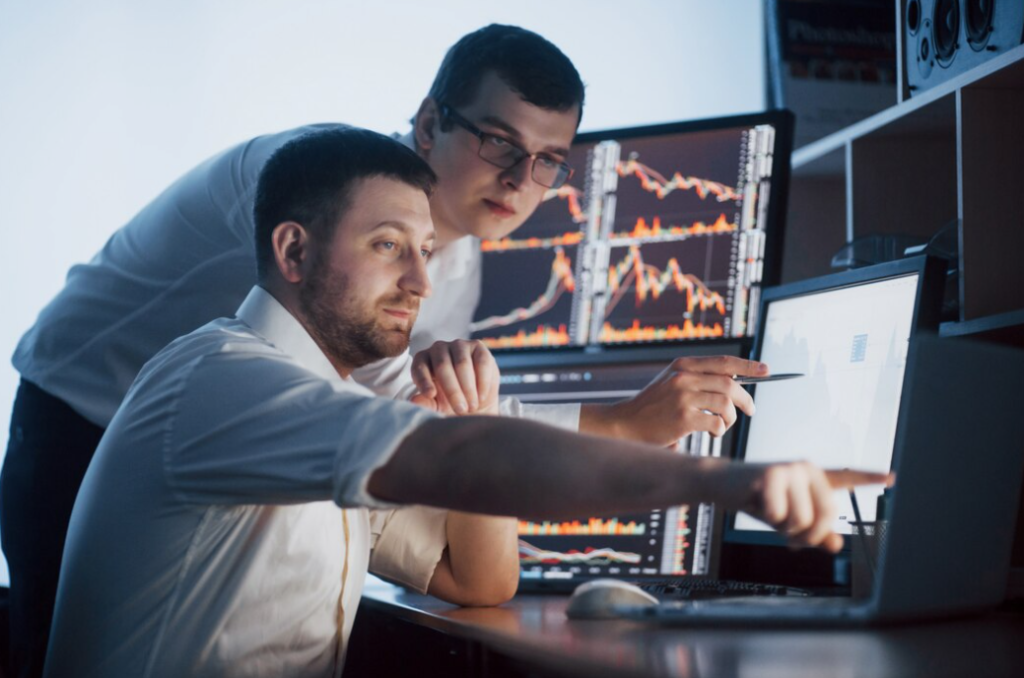
The future of Medium Data is promising and likely to grow in significance as technology continues to advance. With the development of more advanced analytics tools and machine learning algorithms, organizations will extract even greater value from Medium Data sources. Todd Schneider’s work in this field serves as a continued source of inspiration for data professionals, encouraging them to explore the full potential of Medium Data. As diverse data sources emerge and industries increasingly adopt Medium Data, its role in shaping business strategies and decision-making processes will expand. Additionally, ethical considerations and responsible data practices will become even more critical as Medium Data’s use becomes more prevalent. Overall, Medium Data is poised to play a pivotal role in the data-driven landscape of the future.
Conclusion
In conclusion, Todd Schneider’s Medium Data concept bridges the gap between the vastness of Big Data and the simplicity of Small Data. His work exemplifies the power of Medium Data in extracting actionable insights for businesses and industries across the board. As the world becomes increasingly data-centric, embracing the potential of Medium Data is a smart move for those looking to stay competitive and make informed decisions. Todd Schneider’s contributions to this field serve as a testament to the exciting opportunities that Medium Data presents.