Traditionally, risk management relied heavily on intuition, experience, and historical data. However, with the rise of big data and advanced analytics, businesses are shifting towards a more data-driven approach. By leveraging vast amounts of real-time data and powerful analytical tools, organizations can make better-informed decisions, identify risks sooner, and respond more effectively.
This shift from traditional methods to data-driven decision-making transforms how companies manage uncertainty, allowing them to mitigate risks and seize new opportunities proactively.
Key Components of Data-Driven Decision Making in Risk Management
Data-driven decision-making in risk management relies on several key components that work together to enhance accuracy, efficiency, and proactive strategies. These components ensure that organizations are equipped to handle risks effectively by leveraging the power of data.
Data Collection and Quality
The quality and relevance of the data collected are the foundation of any data-driven strategy. Risk management means gathering comprehensive data from multiple sources, such as market trends, customer behaviors, operational performance, and external factors like regulatory changes.
Data Analysis Tools and Techniques
Once data is collected, it is next to analyze it using advanced tools and techniques. Risk managers use various data analysis methods, including statistical modeling, machine learning, and predictive analytics, to identify patterns, trends, and potential risk factors.
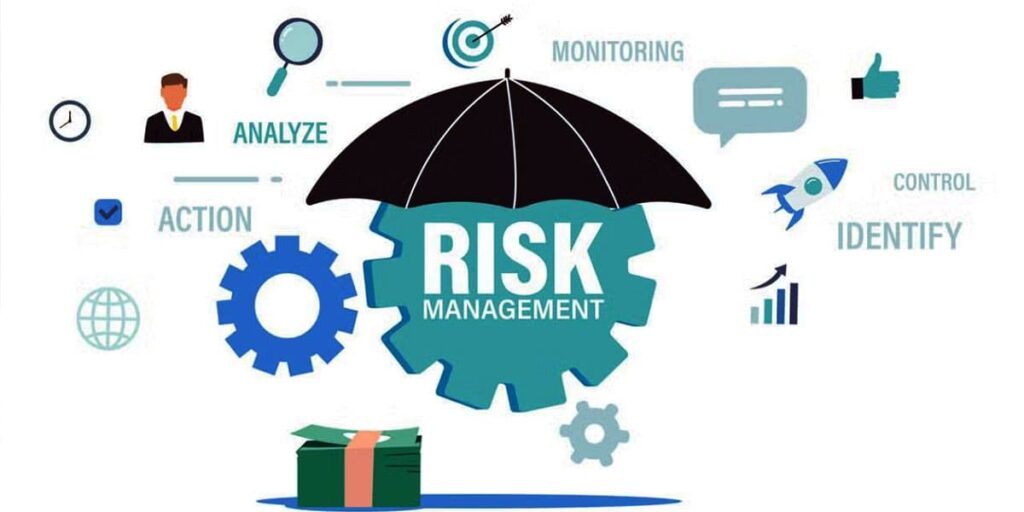
Real-Time Data Processing
Real-time data processing is a game-changer in risk management. Instead of relying on static, historical data, organizations can now access live data streams that reflect current conditions. This enables them to detect emerging risks and respond quickly to changing situations. Real-time monitoring is especially important in industries like finance or cybersecurity, where risks can escalate rapidly.
Predictive Analytics
By analyzing patterns and trends, predictive models can help organizations anticipate risks before they occur, giving them time to prepare and mitigate potential impacts. Predictive analytics identifies possible threats and assesses the likelihood and severity of these risks, enabling more informed decision-making.
Challenges in Data-Driven Risk Management
While data-driven risk management offers significant benefits, it also comes with several challenges that organizations must address to leverage its potential fully. These challenges can stem from the complexities of managing large volumes of data to ensure data privacy and compliance with regulations.
Data Privacy and Security
One of the most pressing concerns in data-driven risk management is maintaining the privacy and security of sensitive information. With increasing data collection comes the responsibility to protect this data from breaches and misuse. Organizations must comply with various data protection regulations, such as GDPR, which require stringent measures to safeguard personal and financial data.
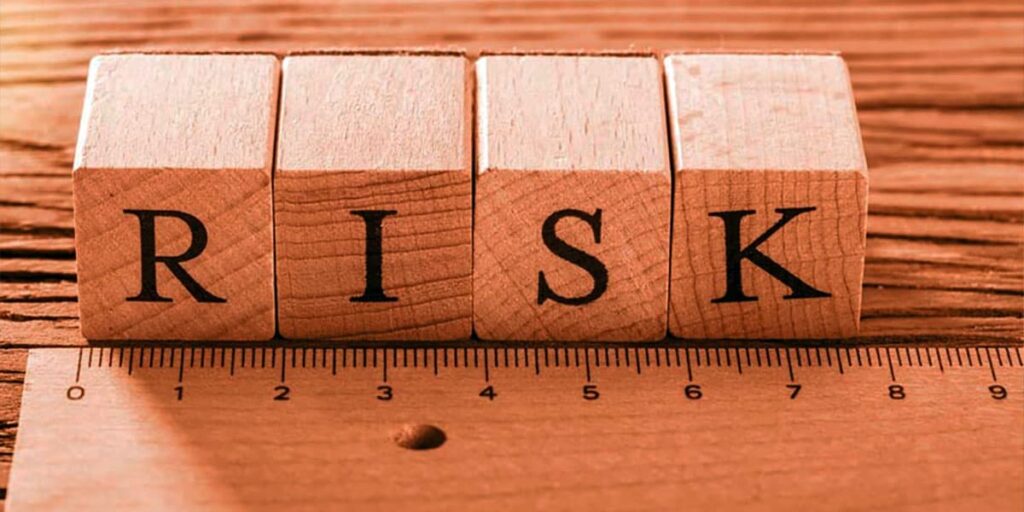
Data Overload and Complexity
The sheer volume and complexity can become overwhelming as businesses gather more data. Not all collected data is relevant to risk management, and organizations must differentiate between useful and unnecessary data. Additionally, managing and processing large datasets requires advanced tools and technical expertise.
Data Accuracy and Reliability
The effectiveness of data-driven decision-making relies heavily on the accuracy and reliability of the data being analyzed. Poor data quality can lead to incorrect risk assessments, negatively impacting decision-making and leading to inadequate risk management outcomes. Ensuring data accuracy requires regular validation, cleansing, and updating datasets to reflect the latest information.
Skilled Personnel and Expertise
Adopting a data-driven risk management approach requires skilled personnel to understand risk management principles and advanced data analysis techniques. Finding professionals who can bridge the gap between data science and risk management can be challenging, especially as the demand for data analysts and risk management experts grows.
Cost and Resource Requirements
Implementing data-driven systems for risk management can be resource-intensive. From acquiring the right technology platforms to hiring skilled personnel, the costs associated with building and maintaining a data-driven infrastructure can be significant, especially for smaller organizations. Additionally, real-time data processing and advanced analytics tools often require substantial investment in IT infrastructure and ongoing maintenance.
Closing Remarks
Data-driven decision-making is transforming the way organizations manage risks, offering deeper insights, real-time monitoring, and predictive capabilities that were previously unattainable. By embracing advanced data analysis, algorithmic audits, and predictive tools, businesses can proactively identify and mitigate risks, ultimately protecting themselves from unforeseen challenges. While there are challenges to implementing this approach, the benefits far outweigh the costs, allowing companies to stay agile and make informed decisions in an increasingly complex risk landscape.